Biography
Valentina's academic career began with a strong foundation in mathematics from St. Petersburg University in Russia, followed by a PhD in Statistics from Cardiff University. Since then, her innovative approaches in statistical methodology have significantly advanced our understanding of Alzheimer's disease.
As a main member of the international Alzheimer’s disease consortia (International Genomics of Alzheimer’s Project consortium (IGAP) and European Alzheimer's Disease DNA BioBank (EADB)), she played a crucial role in identifying new genetic loci linked to Alzheimer's, adding to the knowledge base necessary for developing better diagnostic tools and treatments.
Valentina’s extensive multidisciplinary expertise spans genetic data analysis in dementias including Parkinson’s disease, Vascular dementia, Frontotemporal dementia, Amyotrophic Lateral Sclerosis, and dementia-related phenotypes.
Her work focuses on accurately identifying individuals at high risk of disease, utilising polygenic risk scores, biomarkers and environmental risk factors. Her primary interest, however, remains statistical methodology, and she continually strives to optimise approaches and explore novel methodologies for the analysis of large complex datasets.
Her current objectives include leveraging artificial intelligence (AI) and machine learning methods to enhance disease risk prediction and patient stratification in large population-level data, as well as employing cutting-edge federated and swarm learning approaches for remote and secure data analyses.
Escott-Price Lab
Explore the work of the Escott-Price Lab focused on using Big Data to accelerate discoveries into dementia.
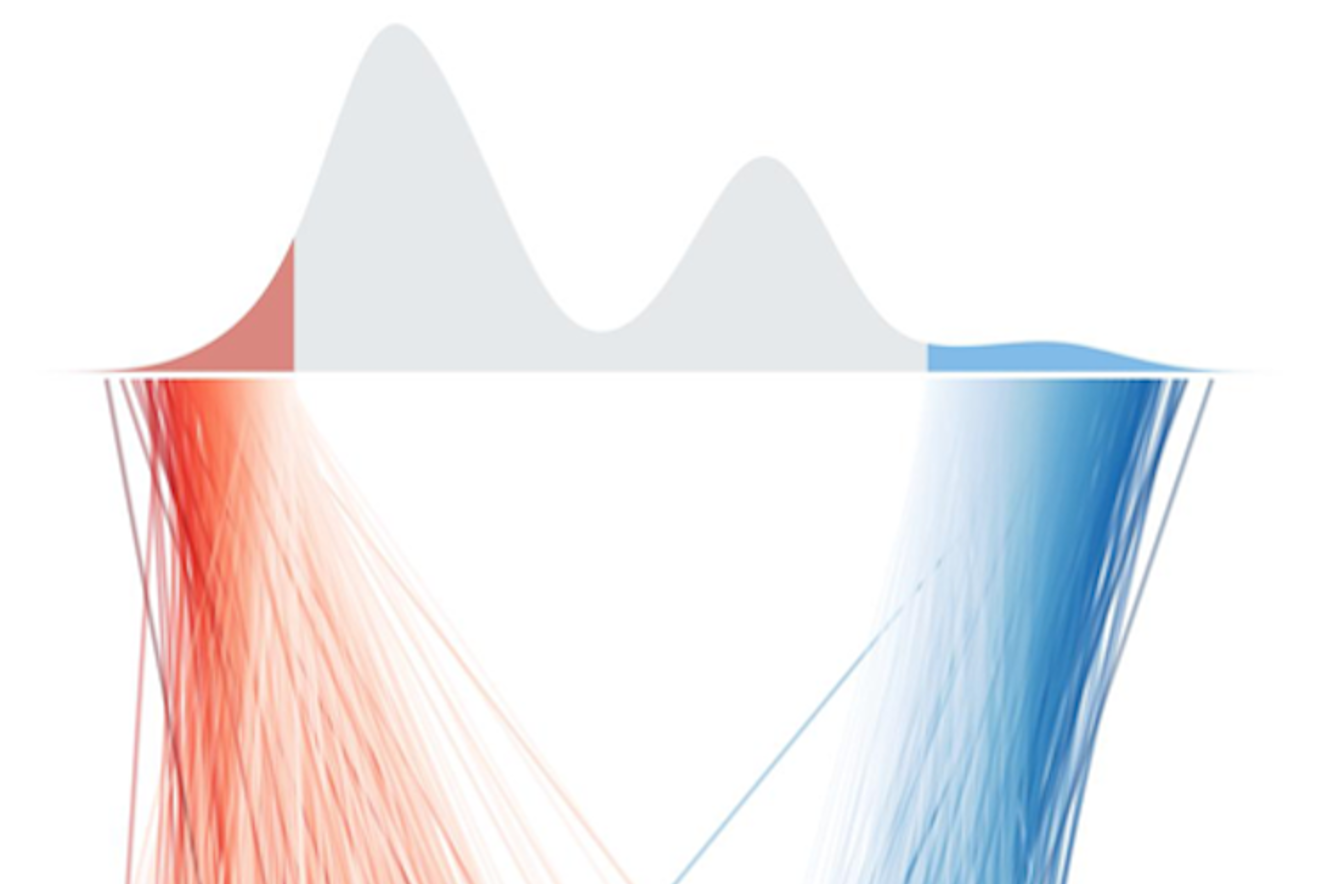