Abstract
medRxiv [Preprint]. 2024 May 31:2024.05.31.24308264. doi: 10.1101/2024.05.31.24308264.
ABSTRACT
Tau positron emission tomography (PET) is a reliable neuroimaging technique for assessing regional load of tau pathology in the brain, commonly used in Alzheimer's disease (AD) research and clinical trials. However, its routine clinical use is limited by cost and accessibility barriers. Here we explore using machine learning (ML) models to predict clinically useful tau-PET outcomes from low-cost and non-invasive features, e.g., basic clinical variables, plasma biomarkers, and structural magnetic resonance imaging (MRI). Results demonstrated that models including plasma biomarkers yielded highly accurate predictions of tau-PET burden (best model: R-squared=0.66-0.68), with especially high contribution from plasma P-tau217. In contrast, MRI variables stood out as best predictors (best model: R-squared=0.28-0.42) of asymmetric tau load between the two hemispheres (an example of clinically relevant spatial information). The models showed high generalizability to external test cohorts with data collected at multiple sites. Based on these results, we also propose a proof-of-concept two-step classification workflow, demonstrating how the ML models can be translated to a clinical setting. This study reveals current potential in predicting tau-PET information from scalable cost-effective variables, which could improve diagnosis and prognosis of AD.
PMID:38853877 | PMC:PMC11160861 | DOI:10.1101/2024.05.31.24308264
UK DRI Authors
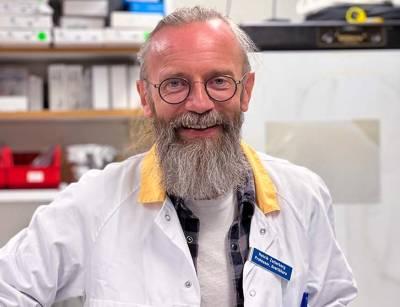