Abstract
Alzheimers Dement. 2025 Feb;21(2):e14600. doi: 10.1002/alz.14600.
ABSTRACT
INTRODUCTION: Tau positron emission tomography (PET) is a reliable neuroimaging technique for assessing regional load of tau pathology in the brain, but its routine clinical use is limited by cost and accessibility barriers.
METHODS: We thoroughly investigated the ability of various machine learning models to predict clinically useful tau-PET composites (load and laterality index) from low-cost and non-invasive features, for example, clinical variables, plasma biomarkers, and structural magnetic resonance imaging (MRI).
RESULTS: Models including plasma biomarkers yielded the most accurate predictions of tau-PET burden (best model: R-squared = 0.66-0.69), with especially high contribution from plasma phosphorylated tau-217 (p-tau217). MRI variables were the best predictors of asymmetric tau load between the two hemispheres (best model: R-squared = 0.28-0.42). The models showed high generalizability to external test cohorts with data collected at multiple sites. Through a proof-of-concept two-step classification workflow, we also demonstrated possible model translations to a clinical setting.
DISCUSSION: This study highlights the promising and limiting aspects of using machine learning to predict tau-PET from scalable cost-effective variables, with findings relevant for clinical settings and future research.
HIGHLIGHTS: Accessible variables showed potential in estimating tau tangle load and distribution. Plasma phosphorylated tau-217 (p-tau217) and magnetic resonance imaging (MRI) were the best predictors of different tau-PET (positron emission tomography) composites. Machine learning models demonstrated high generalizability across AD cohorts.
PMID:39985487 | DOI:10.1002/alz.14600
UK DRI Authors
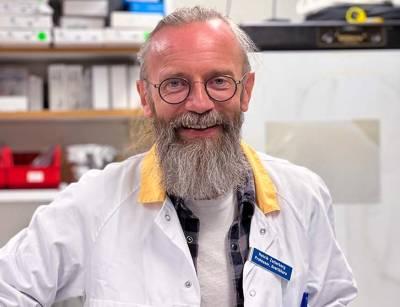