Key details
Using Big Data, machine learning and AI to accelerate discoveries into dementia
Our genes play a key role in determining our risk of developing different diseases. While faults in certain high-risk genes cause rare inherited forms of specific dementia (e.g. Alzheimer’s disease, amyotrophic lateral sclerosis), scientists have also identified many low-risk genetic variations that can increase the likelihood of developing theses neurodegenerative conditions.
Building on these genetic discoveries, scientists are now investigating the functional effects of Alzheimer’s risk genes through laboratory studies and large-scale clinical studies involving thousands of people. These studies aim to understand how these genes interact with environmental and lifestyle factors.
At the UK DRI, the Escott-Price Lab is at the forefront of deploying groundbreaking computational approaches, such as machine learning and artificial intelligence models, to study disease mechanisms across several neurodegenerative disorders. By decoding the complex relationships in large-scale genetic and clinical data, the team aims to reveal how specific genes, gene networks and clinical information predict the onset and progression of dementia and dementia related disease and disorders. This research not only enhances the group's scientific understanding but will ultimately revolutionise personalised medicine approaches, potentially leading to more effective prevention and treatment strategies for neurodegenerative diseases.
Valentina Escott-Price discusses the significance of polygenic risk scores and the current landscape of genomic profiling for the prediction of Alzheimer's disease risk at the Alzheimer’s Research UK Conference 2019
Latest news
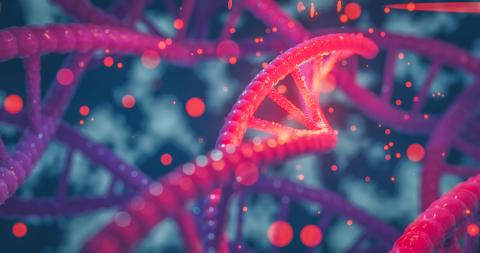
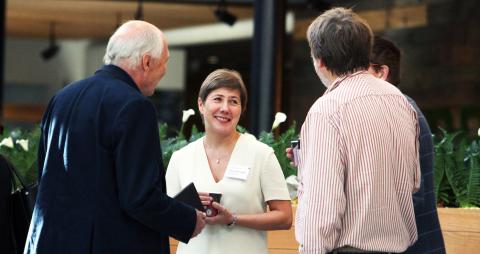
Prof Valentina Escott-Price
Prof Valentina Escott-Price is a Group Leader at the UK DRI at Cardiff. Find out more about her career and expertise on her profile page.
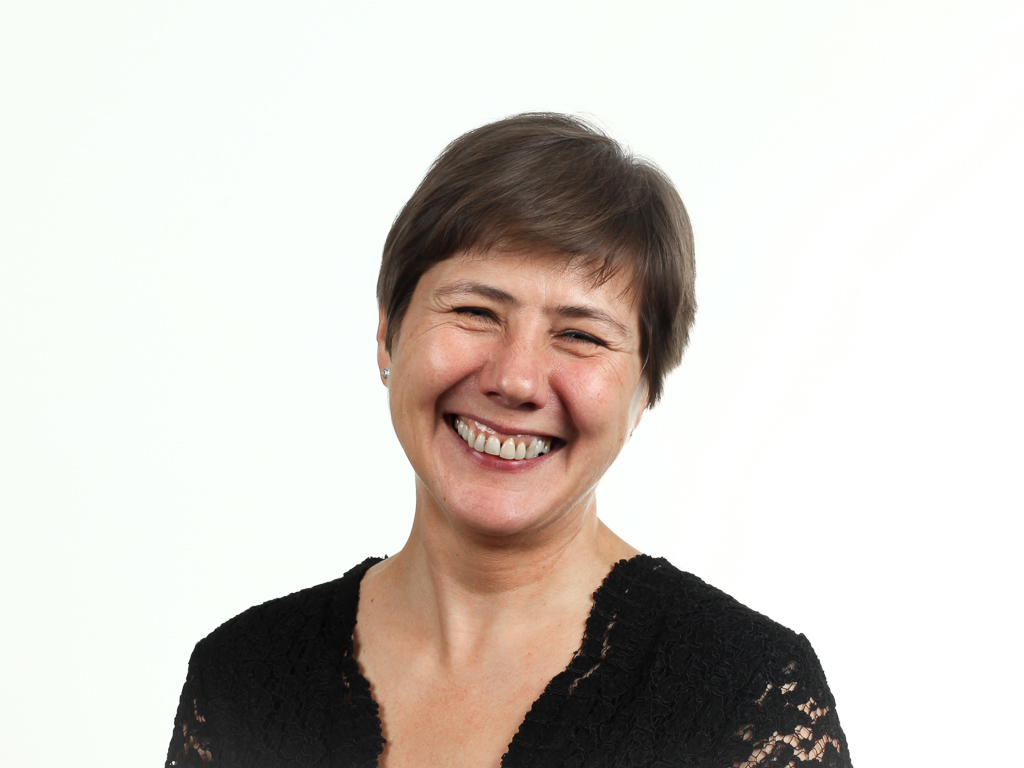
Research summary
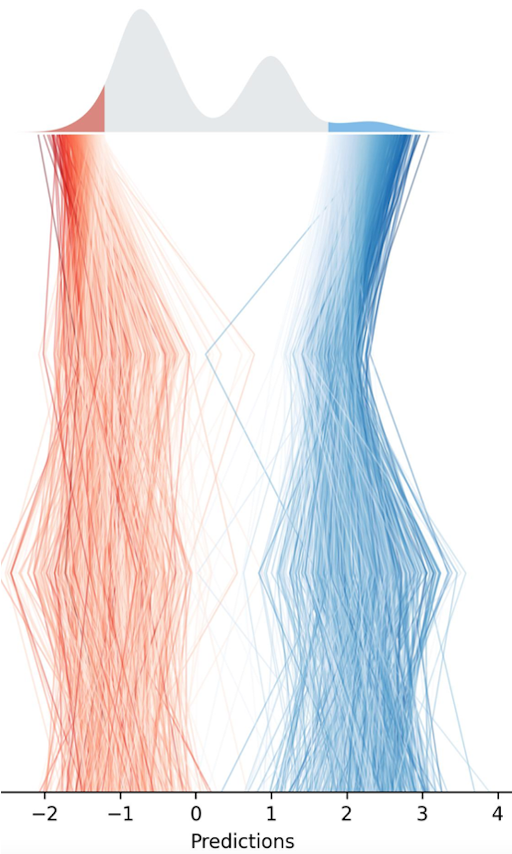
The Escott Price Lab study polygenic risk score in neurodegenerative conditions
Machine learning and AI as tools to identify novel associated genetic risk variants, improve risk prediction and enhance patient stratification
Genome-wide association studies (GWAS) of neurodegenerative disorders (such us Alzheimer’s disease (AD), Parkinson’s disease, Frontotemporal dementia, Vascular dementia, etc.) have identified numerous loci containing common variant risk alleles, paving the way for a deeper understanding of disease biology and designing novel therapies. However, the causal genes, pathways, and processes remain to be fully elucidated.
Translating GWAS findings into biological insights presents several major challenges. Specifically, index GWAS variants are often in linkage disequilibrium (LD) with many other single nucleotide variants (SNPs), any of which could be the causal variant(s). Additionally, there is substantial evidence that most causal alleles reside in non-coding regions of the genome, complicating accurate annotation and functional interpretation. Non-coding elements are often associated with genes over large chromosomal distances and in a cell type-specific manner, further obscuring the identity of true AD risk genes.
The common variant risk for dementia, like other complex disorders, is highly polygenic. The Escott-Price Lab has recently elucidated the significant polygenic component of AD, showcasing its predictive utility for AD risk. This breakthrough is a valuable research tool for enhancing experimental designs, including preventative clinical trials, stem cell selection, and high/low-risk clinical studies. Recent evidence also shows that common transcriptional mechanisms operate across risk loci, indicating that polygenic risk resides in specific transcriptional networks.
Current diagnostic categories do not directly map onto underlying biology and conflict with the continuous nature of many disease phenotypes. Evidence suggests shared genetic risk across neurodegenerative disorders and genetic strata within disorders. Therefore, incorporating phenotyping measures to enhance prediction models of AD, other dementias and subphenotypes is of high interest.
The improvement of current methodologies is vital to progress with the identification of individuals at high risk of disease. The group is at the forefront of utilising machine learning and artificial intelligence approaches in neurodegenerative disorders to identify novel associated genetic risk variants, improve risk prediction and enhance patient stratification.
Prof Escott-Price is currently pioneering a project on federated and swarm learning, approaches which are crucial for handling sensitive genetic data and massive EHRs. These cutting-edge approaches eliminate the need for data sharing across separate servers through Federaled Learning technology. Using deep learning and gradient boosting, they promise to integrate data from more diverse and smaller populations, enhancing genetic studies' inclusivity and robustness. This innovation places the lab at the forefront of secure, collaborative data analysis, paving the way for groundbreaking discoveries in neurodegenerative diseases.
Key publications
Vacancies
Lab members
- Dr Federico Melograna (Postdoctoral Researcher)
- Emily Simmonds (Research Associate)
- Matthew Bracher-Smith (Research Associate)
- Ganna Leonenko (Research Associate)
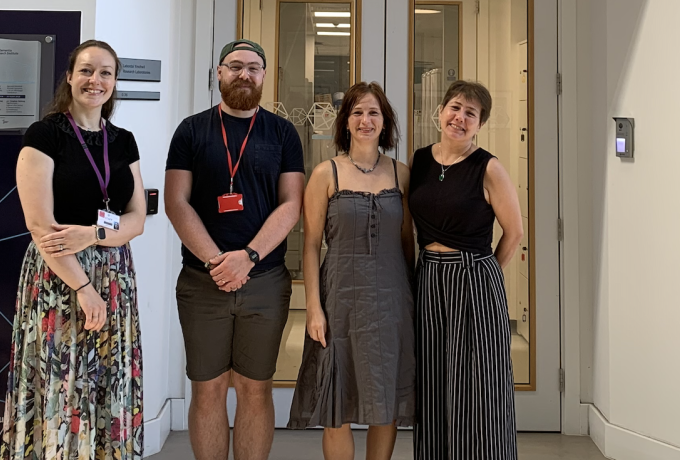
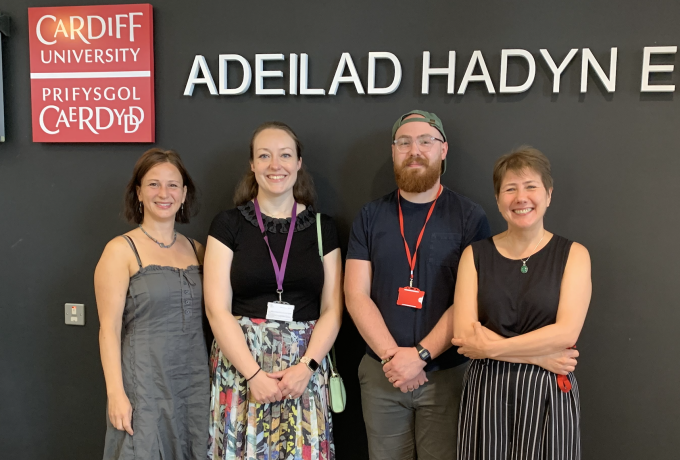
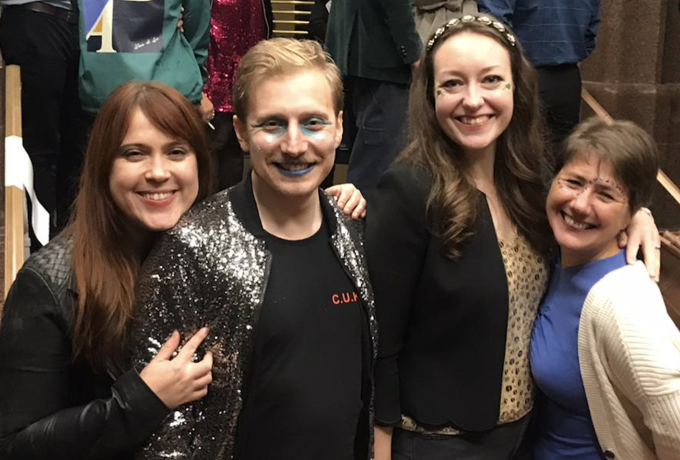
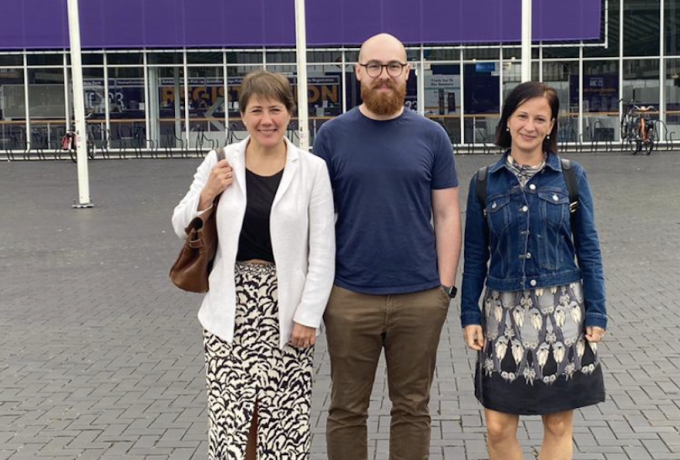
Collaborators
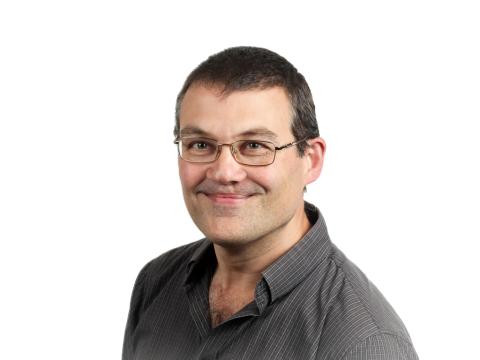
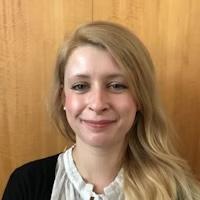
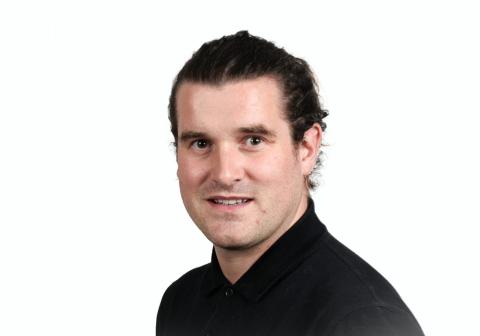
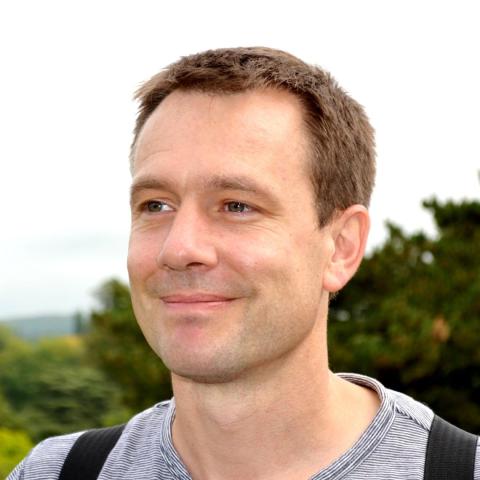
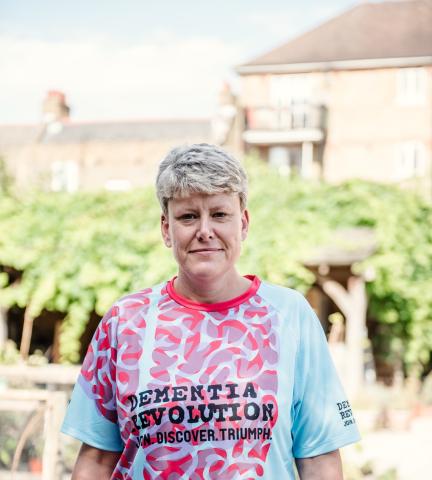
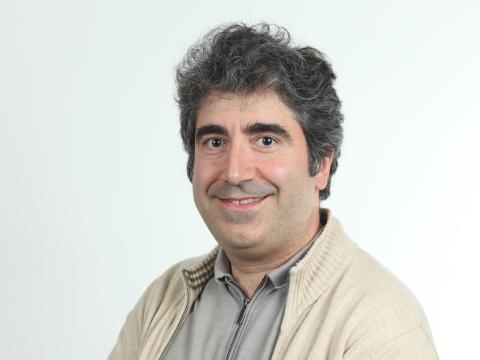
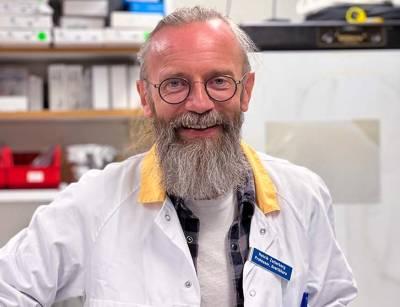
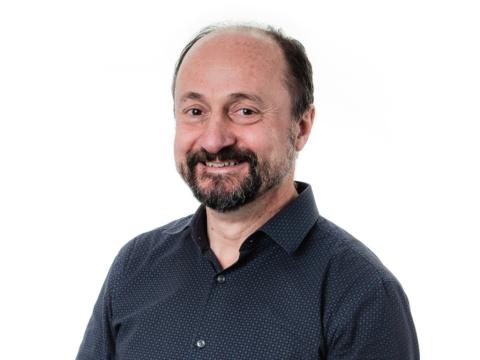
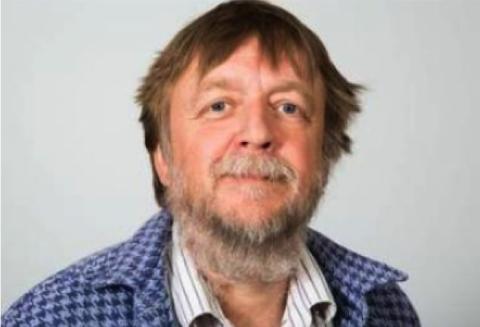
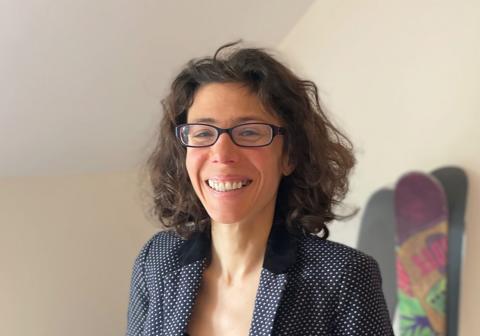
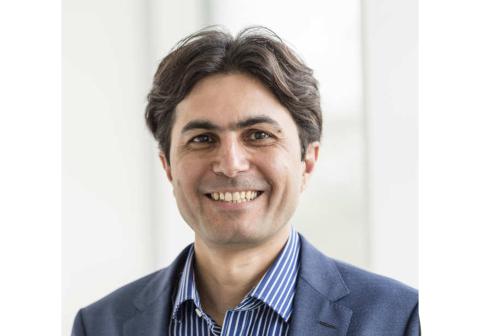
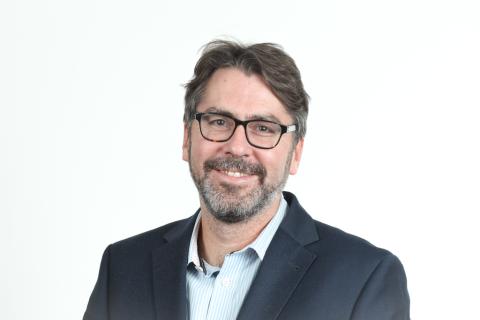
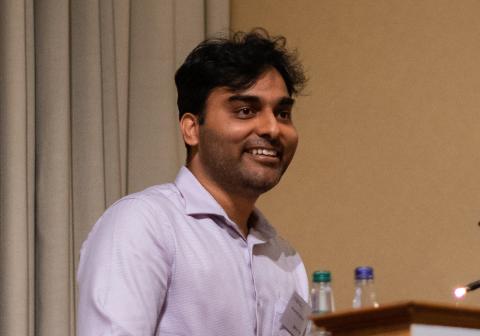
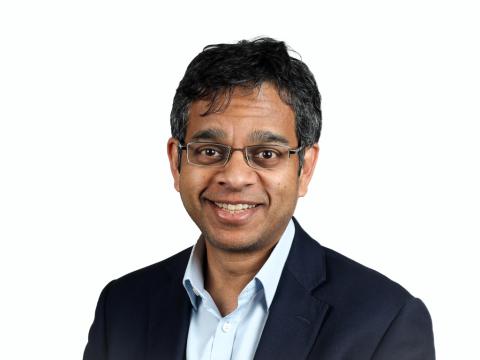
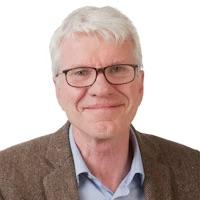
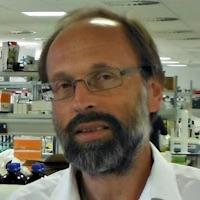
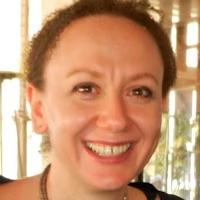
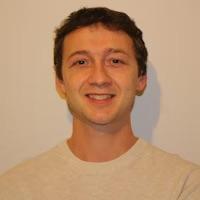
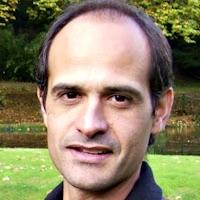
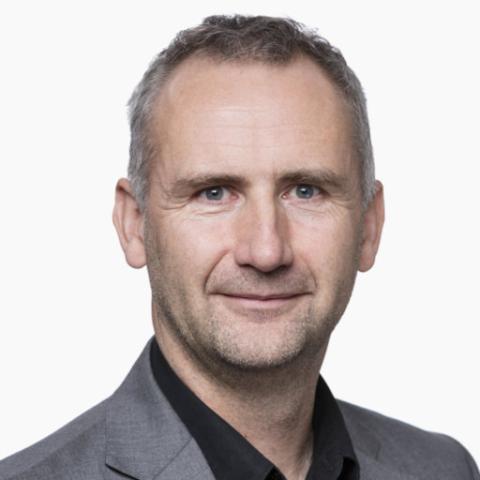
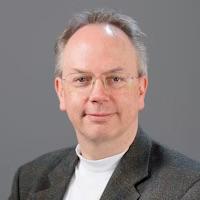
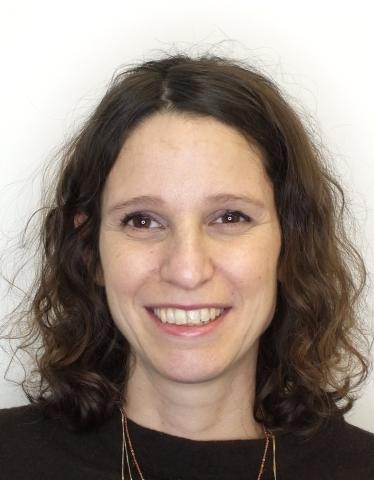
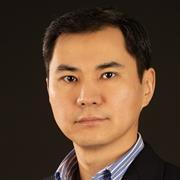
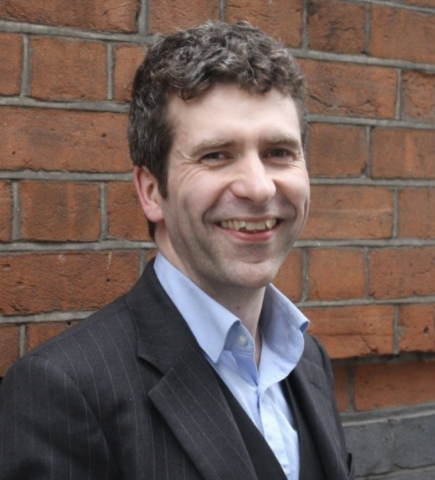
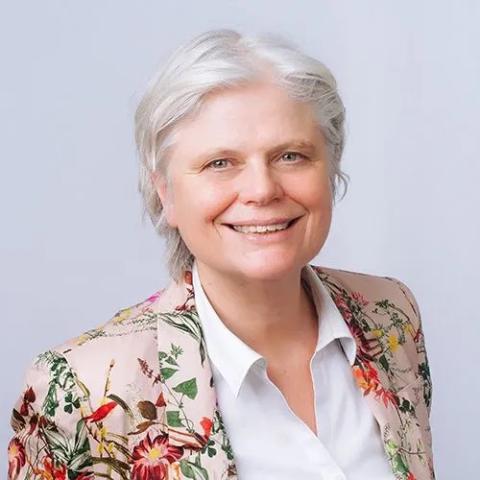
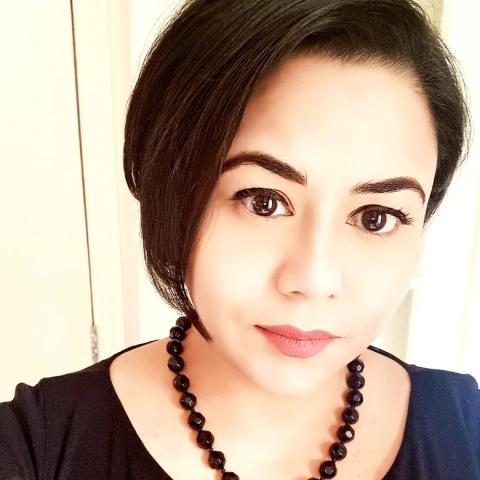
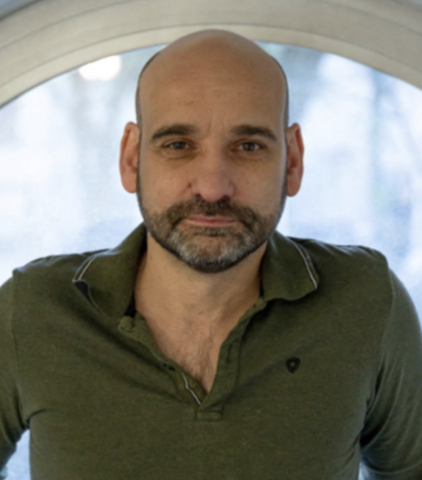
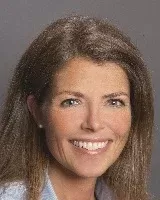
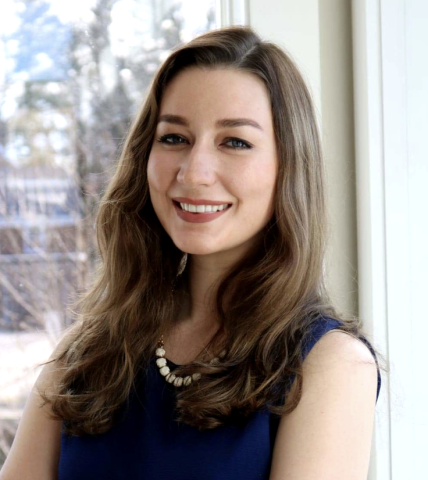
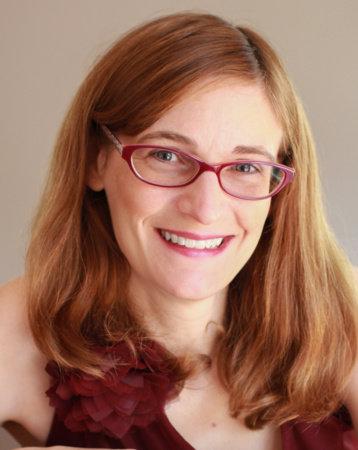
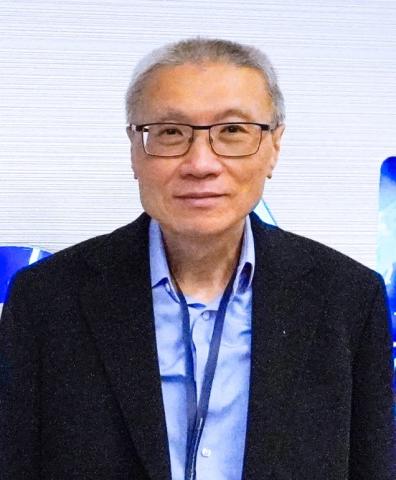
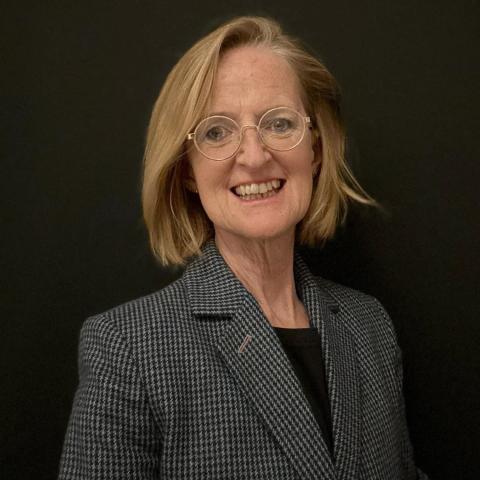
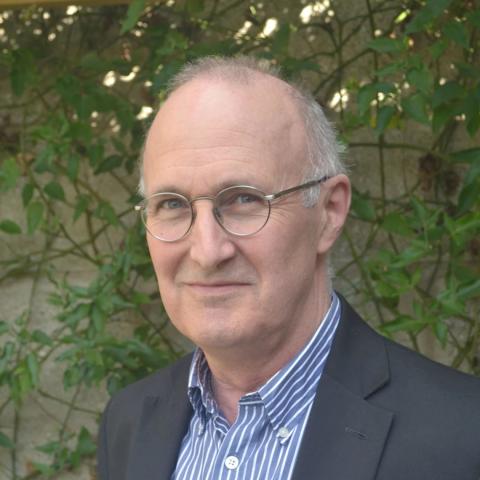
Lab funders
Thank you to all those who support the Escott-Price Lab!
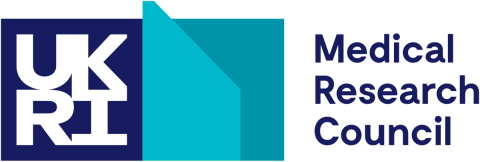